Yesterday the silicon chip platform company Nvidia became the most valuable public company in the US, surpassing Microsoft:
Nvidia became the U.S.’s most valuable listed company Tuesday thanks to the demand for its artificial-intelligence chips, leading a tech boom that brings back memories from around the start of this century.
Nvidia’s chips have been the workhorses of the AI boom, essential tools in the creation of sophisticated AI systems that have captured the public’s imaginationwith their ability to produce cogent text, images and audio with minimal prompting. (Wall Street Journal)
The buzzy hype around Nvidia and AI generally is feeling very dot-com-bubbly, for sure, but there’s likely to be some truth in CEO Jenson Huang’s claim:
“We are fundamentally changing how computing works and what computers can do,” Mr. Huang said in a conference call with analysts in May. “The next industrial revolution has begun.” (New York Times)
An earlier discussion of that May earnings call in Yahoo Finance is also informative. Huang is right that computing in the blossoming AI era will be qualitatively different from the past five decades. and those changes are showing up in forecasts of increased investment in data centers.
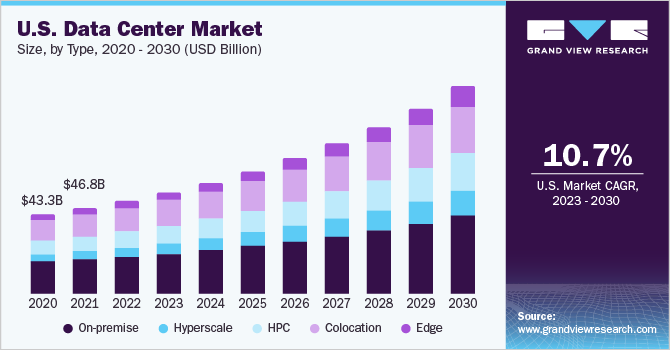
Past computing relied on CPUs (central processing units). These processors perform calculations based on instructions, and have advanced to do so at rapid rates; innovations symbolized by Moore’s Law have meant that more of these processors can fit in smaller chips, so more and faster computing capability per square inch of space inside the case. But the chips that Nvidia pioneered in the 1990s had different uses and different architecture. These GPUs (graphical processing units) were originally designed for graphics in video games, an industry that they transformed. What made them capable of delivering high quality dynamic graphics was their parallel architecture, their ability to perform many independent calculations at the same time and the same speed to turn 3D images into better and better 2D representations that could move and change dynamically.
It turns out that this massively parallel chip architecture is actually a general purpose technology and not just a graphics processing technology, as Huang and his colleagues saw through the 2000s, building a coding platform and a developer ecosystem around their GPUs. Nvidia has evolved from a GPU company to a machine learning company to an AI company. These capabilities are what drive AI training and, once the model is trained, the ongoing inference that the model performs to deliver answers to users.
If you are at all interested in this rich history, in the history of technology, and/or the history of Nvidia as a company, I cannot recommend highly enough the Acquired podcast three-part series on Nvidia’s history (Part 1) (Part 2) (Part 3), as well as their interview with Jenson Huang in 2023, after the November 2022 AI takeoff.
All of this computing requires energy. Chip manufacturers, Nvidia included, have generally designed chips to minimize energy use per calculation, but they still require energy and still generate waste heat. The way CPUs work and the density of their use in the space meant that data centers could work, physically and economically, while air-cooled. Air cooling meant increased electricity demand from air conditioning as computation and data centers grew. But AI training uses GPUs with their greater capabilities and commensurately greater energy requirements, both for the greater computation load and for cooling (more on that topic in a future post).
In a 2020 Science article, researcher Eric Masanet and co-authors analyzed the growth in data center energy use and improvements in data center energy efficiency in the 2010s, before the AI era:
Since 2010, however, the data center landscape has changed dramatically (see the first figure). By 2018, global data center workloads and compute instances had increased more than sixfold, whereas data center internet protocol (IP) traffic had increased by more than 10-fold (1). Data center storage capacity has also grown rapidly, increasing by an estimated factor of 25 over the same time period. …
But since 2010, electricity use per computation of a typical volume server—the workhorse of the data center—has dropped by a factor of four, largely owing to processor efficiency improvements and reductions in idle power. At the same time, the watts per terabyte of installed storage has dropped by an estimated factor of nine owing to storage-drive density and efficiency gains. (Masenet et al. 2020)
As researcher Alex deVries noted in his 2023 paper in Joule, AI electricity requirements could soon be as large as a country and the energy requirements of the GPU chips are substantial (deVries 2023) (New York Times).
This is why the topic du jour in electricity is data center electricity demand and its implications for infrastructure systems that are very slow to change.
For most of the past 60 years the demand for electricity has grown at a pretty steady rate. The average annual growth rate of electricity consumption in the US over the past 60 years has been approximately 2.2%, with a significant increase in electricity use from about 700 billion kWh in 1960 to over 4 trillion kWh in recent years (EIA Energy). Within that broad trend the growth rate has varied over different periods, with more rapid increases in earlier decades and slower growth in recent years due to improved energy efficiency, changes in the economy, and shifts towards renewable energy sources.
The recent demand growth narrative in electricity has been the decrease in 2008 due to the financial crisis; after a rebound demand again grew at a low rate. Between 2008 and 2019, electricity consumption growth was relatively flat, with an overall average annual growth rate of about 0.3% (EIA Energy).
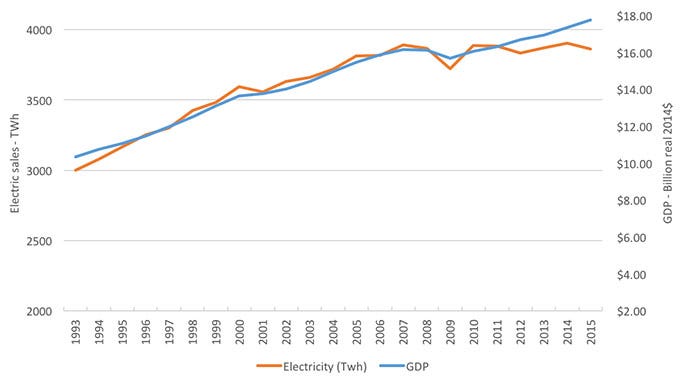
Source: ACEEE 2016
The economics and the business and regulatory models for the electricity industry are predicated on slow, steady growth, so stagnant demand 2008-2019 caused utilities and their shareholders and bankers considerable concern. Then the pandemic happened. During the early stages of the pandemic, particularly in the second quarter of 2020, there was a notable decline in overall electricity consumption, due primarily to reduced economic activity and widespread lockdowns leading to a decrease in industrial and commercial electricity use and a shift to residential consumption (Cicala 2023). The Federal Energy Regulatory Commission reported a drop in electricity demand by approximately 3.5% during this period compared to the same timeframe in 2019.
Fast forward to 2023. In November 2022 OpenAI had published their ChatGPT large language model (LLM) AI, and in the following weeks and months other companies (Anthropic’s Claude, Google Gemini, among others) published their models. ChatGPT reached one million users within just five days of its launch, making it the fastest-growing consumer application in history. By February 2023, it had surpassed 100 million monthly active users. The growth in Nvidia’s stock valuation is a good reflection of the potential magnitude of these AI breakthroughs (and it’s reflected in Microsoft’s and Apple’s too):
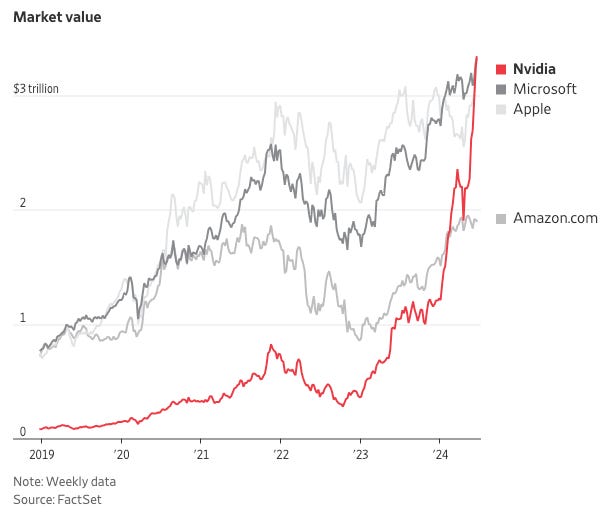
Source: Wall Street Journal 2024
As we’ve been hearing for the past year, AI computation requires considerably more processing and electricity than previous types of computing. We already produce and process enormous amounts of data, and chip architects and data center designers have been attuned to computation’s energy use for decades. In 2019, data centers accounted for approximately 2% of total electricity consumption in the United States. Date centers are energy-intensive, consuming 10 to 50 times more energy per square foot than typical commercial office buildings. In the early 2000s, data centers saw rapid growth in electricity use, with annual increases of around 24% from 2005 to 2010. This growth rate then slowed to about 4% annually from 2010 to 2014, and it continued at this rate up to 2020 (Lawrence Berkeley National Lab).
That pattern is changing, and changing quickly. 2024 is the year when utility forecasts and investment plans are dominated by data center growth (S&P Global Market Intelligence). Previous 5-year and 10-year demand forecasts are now obsolete and utilities and grid operators are revising their forecasts, challenged by the extent to which the AI data center is a qualitatively different cause of electricity demand, one with substantial productivity repercussions through the economy. Forecasting is always difficult, especially about the future (thank you Yogi Berra), but it’s even more difficult when the changes are deep technological ones that make your historical data even less of a guide for future expectations.
A recent Grid Strategies report (Grid Strategies 2023) analyzed the implications of data center electricity demand, and the numbers are staggering for an industry whose business model is predicated on slow and steady demand growth. These Summer 2028forecasts (in gigawatts, that’s 1 million kilowatts) of peak demand are striking in how they changed from 2022 to 2023:
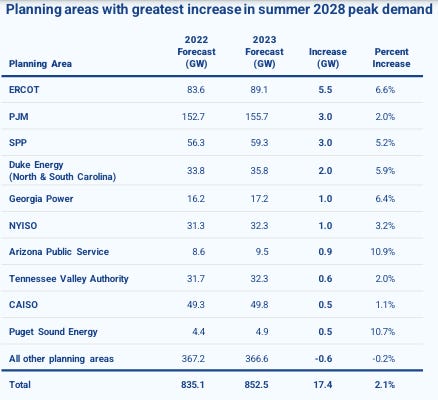
Source: Grid Strategies 2023
This updated forecast from PJM, the grid operator for the mid-Atlantic through Illinois and the largest grid operator in the US, indicates how the large and sudden growth in data center electricity demand is forcing them to revise their forecasts:
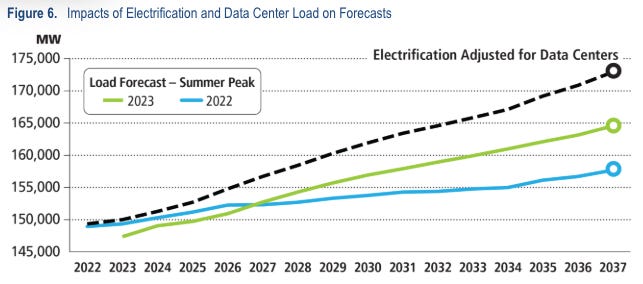
Source: PJM, Energy Transition in PJM, 2023
Such uncertainty is a substantial challenge, technologically, economically, politically, and culturally within the industry.
In this post I simply want to frame the problem facing the electricity industry and its regulators and the data center industry. Over the next couple of weeks I’m going to tease apart some pieces of this problem, bring in some economics insights that I hope will contribute to our understanding of the problem and some implications we can draw for better policy.
This piece was originally published on Lynne’s substack, Knowledge Problem. If you enjoyed the piece, please consider subscribing here.